Biomedical
Wearable Smart Sensors and sensor fusion for Biomedical Applications
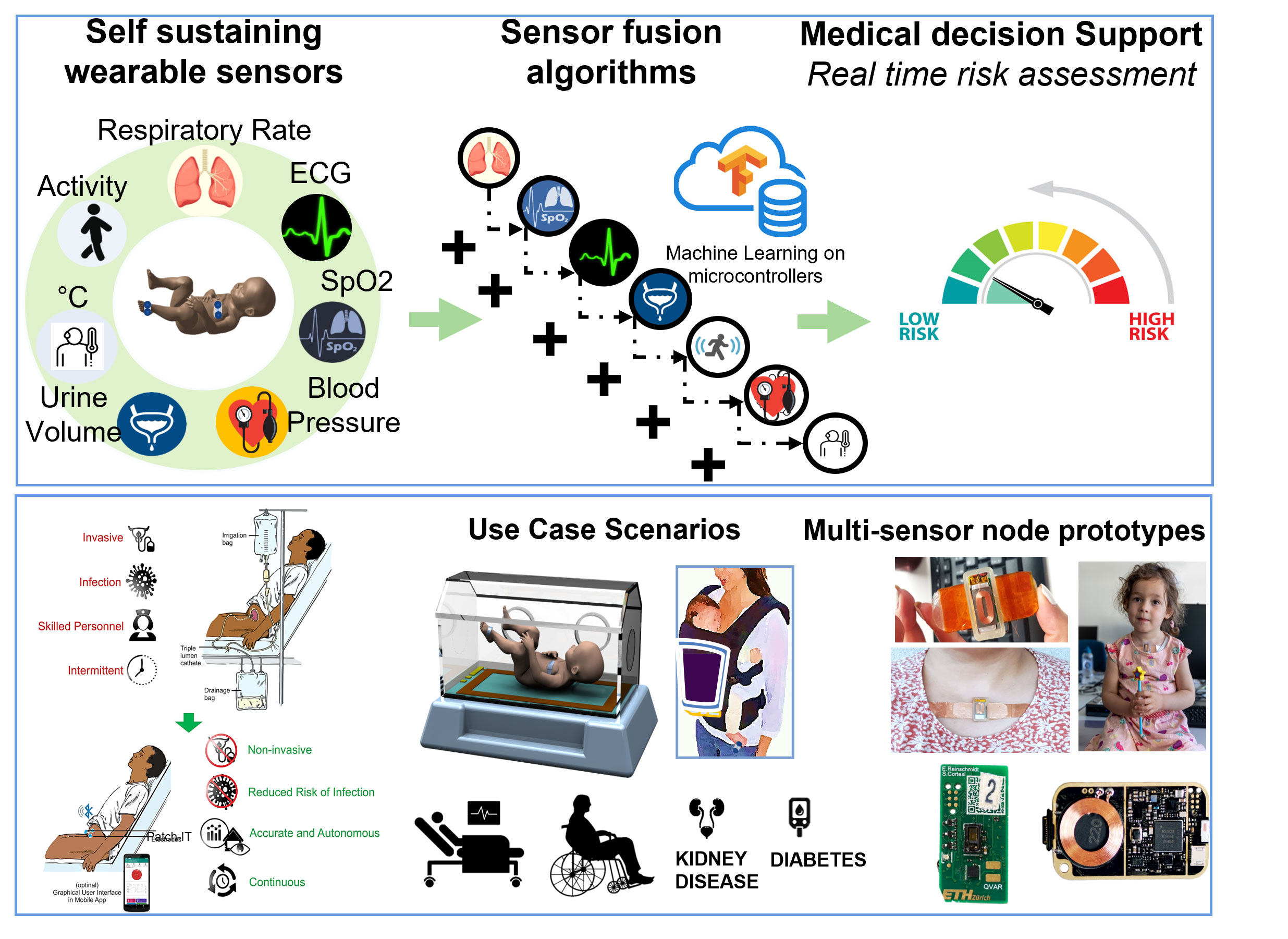
Efficient patient monitoring and management are crucial for better patient outcomes, especially in vulnerable population groups such as, the neonatal intensive care unit, paraplegic care, geriatric care or monitoring of chronic conditions such as disbetes, hypertension or chronic kidney disease. Current monitoring platforms are sporadic (out patient settings) or attach multiple wires with aggressive adhesives in only the intensive care units of hospitals. Even in developed countries, the parameters from the patient monitors are periodically updated. This delay coupled with sparse data over time leads to erroneous detection of patient health deterioration. On the other hand, patients of spinal chord injury, the urinary condition is particularly complicated because they generally lack control over urinary function and cannot voluntarily discharge the bladder. Depending on the severity of the spinal cord injury, micturition reflexes are often blocked and patients with impaired or destroyed nervous pathways may exhibit bladder-sphincter dyssynergia, which causes functional outlet obstruction. Typically, these patients have no natural desire to urinate. To discharge their bladder, paraplegic patients have to manually activate spinal micturition reflexes or use intermittent catheterization which (on the long- term) can cause injury, infection or kidney damage, in addition to other complication in health. Particularly for this paraplegic population, proper monitoring of the bladder volume is very important. At present, no optimum solution present commercially or in published research that can provide a method for accurate, long term, non-invasive and unobtrusive bladder monitoring.
We propose a unique solution, PATCH-IT: a multivital sign monitoring system to assist in diagnostics by continuously monitoring multiple vital signs to aid in disease management of sepsis ( neonatal and adult) patients with digital biomarkers. Our approach would result in providing medical decision support using self-sustainable multi-sensor smart patch with on-board tiny machine learning based personalized medicine algorithms in a mesh enabled IoT setup enabling accurate alerts for clinical deterioration. Autonomous, low power and mesh enabled multi-sensor wearable systems based on the always-on smart sensing paradigm can continuously acquire, process and stream physiological data in real time to a common database and are trained to autonomously detect early and late onset of neonatal sepsis symptoms. Thus eliminating delays in electronic medical records and reducing alarm fatigue.
The main objectives addressed with this research are:
a) Development a self-sustainable and user friendly multi-sensor smart patch that can be as attached to the body for long periods. Investigation of system performance and usability of wearable ECG, PPG, activity, blood pressure, skin impedance and non-invasive urine volume monitoring wearable sensors..
b) Preliminary clinical validation for the implementation of autonomous sensor functionality.
c) Development of algorithms for detection of degradation of patient condition by identifying critical risk factors and digital biomarkers.
d) Large cohort clinical validation of developed multi-sensor patches and personalized models for sepsis identification and risk stratification.