Event-based Sensors
Event-based sensors and sensing, including energy-efficient neuromorphic processing
ColibriES: Neuromorphic Platform for Low-Latency Closed-loop Control
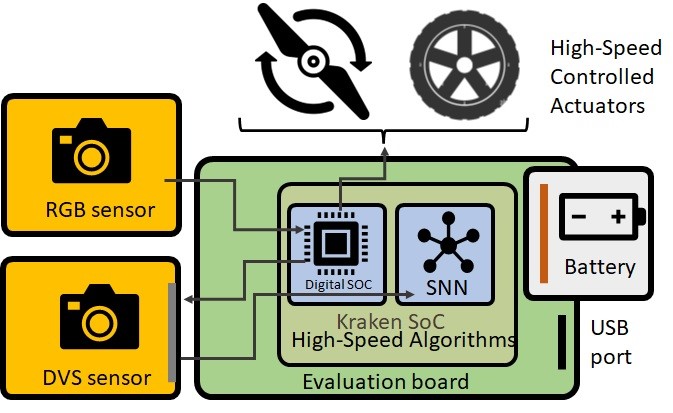
End-to-end event-based computation has the potential to push the envelope in latency and energy efficiency for edge AI applications. Unfortunately, event-based sensors (e.g., DVS cameras) and neuromorphic spike-based processors (e.g., Loihi) have been designed in a decoupled fashion, thereby missing major streamlining opportunities. We presented ColibriES, the first-ever neuromorphic hardware embedded system platform with dedicated event-sensor interfaces and full processing pipelines. ColibriES includes event and frame interfaces and data processing, aiming at efficient and long-life embedded systems in edge scenarios. ColibriES is based on the Kraken system-on-chip and contains a heterogeneous parallel ultra-low power (PULP) processor, frame-based and event-based camera interfaces, and two hardware accelerators for the computation of both event-based spiking neural networks and frame-based ternary convolutional neural networks. We explored and accurately evaluated the performance of event data processing on the example of gesture recognition on ColibriES, as the first step of full-system evaluation. In our experiments, we demonstrate a chip energy consumption of 7.7 mJ and latency of 164.5 ms of each inference with the DVS Gesture event data set as an example for closed-loop data processing, showcasing the potential of ColibriES for battery-powered applications such as wearable devices and UAVs that require low-latency closed-loop control.
Neuromorphic Edge Computing for Biomedical Applications: Gesture Classification Using EMG Signals
With the emergence of edge-computing platforms, the applications of smart wearable devices are immense. This technology can be incorporated in consumer products such as smartwatches and activity trackers, for continuous health monitoring, as well as for medical applications such as myoelectric prosthetics, to interpret the electric activity in the residual limb and achieve fast and precise control. This article presents two spiking neural networks (SNNs) for event-based electromyography (EMG) gesture recognition and their evaluation on Intel’s research neuromorphic chip Loihi. Specifically, the evaluation is done on the Kapoho Bay platform which embeds the Loihi processor in a Universal Serial Bus (USB) form factor device allowing for closed-loop edge computation.
Eye Tracking for Smart Glasses with Event Camera and Neuromorphic Processing
Eye tracking has many potential applications in a large variety of fields, ranging from computer interaction in augmented and virtual reality to human research and safety, for example while driving. To enable more applications and widespread usage, low latency and power consumption is required. Event cameras are well suited for this task with their higher framerate, no motion blur and low power consumption. However, their output is sparse and asynchronous changes in brightness instead of regular images and therefore require novel methods. The two main goals of this project are first to create a dataset for eye tracking at with commercial event based fps and second to develop neuromorphic models running on Intel Loihi and ETH Kraken, train them on the dataset and evaluate their performance, with the aim of achieving low latency and computation suitable for smart glasses.